Course Content
1.
Accuracy Score
0 min
2 min
0
2.
Activation Function
0 min
2 min
0
3.
Algorithm
0 min
2 min
0
4.
Assignment Operator (Python)
0 min
2 min
0
5.
Artificial General Intelligence (AGI)
0 min
3 min
0
6.
Artificial Intelligence
0 min
4 min
0
7.
Artificial Narrow Intelligence (ANI)
0 min
3 min
0
8.
Artificial Neural Network (ANN)
0 min
2 min
0
9.
Backpropagation
0 min
2 min
0
10.
Bias
0 min
2 min
0
11.
Bias-Variance Tradeoff
0 min
2 min
0
12.
Big Data
0 min
2 min
0
13.
Business Analyst (BA)
0 min
2 min
0
14.
Business Analytics (BA)
0 min
2 min
0
15.
Business Intelligence (BI)
0 min
1 min
0
16.
Categorical Variable
0 min
1 min
0
17.
Clustering
0 min
2 min
0
18.
Command Line
0 min
1 min
0
19.
Computer Vision
0 min
2 min
0
20.
Continuous Variable
0 min
1 min
0
21.
Cost Function
0 min
2 min
0
22.
Cross-Validation
0 min
2 min
0
23.
Data Analysis
0 min
7 min
0
24.
Data Analyst
0 min
4 min
0
25.
Data Science
0 min
1 min
0
26.
Data Scientist
0 min
6 min
0
27.
Early Stopping
0 min
2 min
0
28.
Exploratory Data Analysis (EDA)
0 min
2 min
0
29.
False Negative
0 min
1 min
0
30.
False Positive
0 min
1 min
0
31.
Google Colaboratory
0 min
2 min
0
32.
Gradient Descent
0 min
2 min
0
33.
Hidden Layer
0 min
2 min
0
34.
Hyperparameter
0 min
2 min
0
35.
Image Recognition
0 min
2 min
0
36.
Imputation
0 min
2 min
0
37.
K-fold Cross Validation
0 min
2 min
0
38.
K-Means Clustering
0 min
2 min
0
39.
Linear Regression
0 min
2 min
0
40.
Logistic Regression
0 min
1 min
0
41.
Machine Learning Engineer (MLE)
0 min
5 min
0
42.
Mean
0 min
2 min
0
43.
Neural Network
0 min
2 min
0
44.
Notebook
0 min
3 min
0
45.
One-Hot Encoding
0 min
2 min
0
46.
Operand
0 min
1 min
0
47.
Operator (Python)
0 min
1 min
0
48.
Print Function (Python)
0 min
1 min
0
49.
Python
0 min
5 min
0
50.
Quantile
0 min
1 min
0
51.
Quartile
0 min
1 min
0
52.
Random Forest
0 min
2 min
0
53.
Recall
0 min
2 min
0
54.
Scalar
0 min
2 min
0
55.
Snake Case
0 min
1 min
0
56.
T-distribution
0 min
2 min
0
57.
T-test
0 min
2 min
0
58.
Tableau
0 min
2 min
0
59.
Target
0 min
1 min
0
60.
Tensor
0 min
2 min
0
61.
Tensor Processing Unit (TPU)
0 min
2 min
0
62.
TensorBoard
0 min
2 min
0
63.
TensorFlow
0 min
2 min
0
64.
Test Loss
0 min
2 min
0
65.
Time Series
0 min
2 min
0
66.
Time Series Data
0 min
2 min
0
67.
Test Set
0 min
2 min
0
68.
Tokenization
0 min
2 min
0
69.
Train Test Split
0 min
2 min
0
70.
Training Loss
0 min
2 min
0
71.
Training Set
0 min
2 min
0
72.
Transfer Learning
0 min
2 min
0
73.
True Negative (TN)
0 min
1 min
0
74.
True Positive (TP)
0 min
1 min
0
75.
Type I Error
0 min
2 min
0
76.
Type II Error
0 min
2 min
0
77.
Underfitting
0 min
2 min
0
78.
Undersampling
0 min
2 min
0
79.
Univariate Analysis
0 min
2 min
0
80.
Unstructured Data
0 min
2 min
0
81.
Unsupervised Learning
0 min
2 min
0
82.
Validation
0 min
2 min
0
83.
Validation Loss
0 min
1 min
0
84.
Vanishing Gradient Problem
0 min
2 min
0
85.
Validation Set
0 min
2 min
0
86.
Variable (Python)
0 min
1 min
0
87.
Variable Importances
0 min
2 min
0
88.
Variance
0 min
2 min
0
89.
Variational Autoencoder (VAE)
0 min
2 min
0
90.
Weight
0 min
1 min
0
91.
Word Embedding
0 min
2 min
0
92.
X Variable
0 min
2 min
0
93.
Y Variable
0 min
2 min
0
94.
Z-Score
0 min
1 min
0

- Save
- Run All Cells
- Clear All Output
- Runtime
- Download
- Difficulty Rating
Loading Runtime
Clustering in machine learning is a technique used to group similar data points together based on certain characteristics or features. The goal of clustering is to identify natural groupings in the data without any prior knowledge of the group memberships. In other words, it is an unsupervised learning approach where the algorithm tries to find patterns and structures within the data without explicit guidance on what those patterns should be.
The process of clustering involves assigning data points into clusters in such a way that data points within the same cluster are more similar to each other than to those in other clusters. The similarity between data points is typically determined using distance or similarity measures.
There are various clustering algorithms, each with its own approach to defining and forming clusters. Some commonly used clustering algorithms include:
- K-Means Clustering: This algorithm partitions the data into k clusters by iteratively assigning data points to the nearest cluster center and updating the center based on the mean of the assigned points.
- Hierarchical Clustering: This method builds a hierarchy of clusters, starting with individual data points and merging them into larger clusters based on their similarities. It can result in a tree-like structure called a dendrogram.
- DBSCAN (Density-Based Spatial Clustering of Applications with Noise): DBSCAN groups together data points that are close to each other and have a sufficient number of neighbors, while marking isolated data points as outliers.
- Agglomerative Clustering: Similar to hierarchical clustering, agglomerative clustering starts with individual data points and progressively merges them into larger clusters based on a linkage criterion.
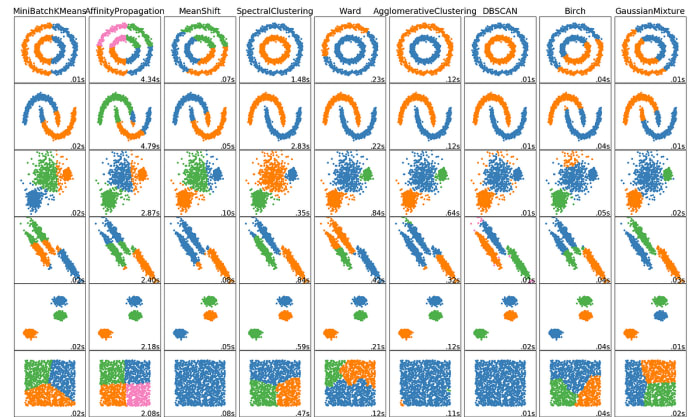
Clustering is widely used in various applications, such as customer segmentation, anomaly detection, image segmentation, and document categorization. The choice of a clustering algorithm depends on the characteristics of the data and the specific goals of the analysis.